Process optimization and analytics for mining
Mining companies report the drive to adapt in a constantly changing market
This is the tooltip content
- Grade decline
- Complex ore bodies
- Complex and remote operations add capital and operational costs
- Environmental regulation
- License to operate
- Sustainable operation
- Population, urbanization, technology and energy driving consumption
- Market volatility as a new normal
- Skill gaps
- New skills required
- Next generation
Model Predictive Control
Basic control strategies aren't enough
Although regulatory control provides adequate control in terms of plant safety, it rarely achieves optimal control in terms of quality, nor does it operate in the most economical fashion.
Model Predictive Control
Click the mining equipment to learn about the benefits of Model Predictive Control
Process Optimization Challenges
- Continuous variability of raw materials
- Frequent internal and external disturbances
- Process delays
- Key variables difficult to measure
- Many recirculating loads and multiple interactions
MPC Benefits
- Increased throughput
- Lower reagent consumption
- Optimum use of water and energy
- Improved process stability
- Better recovery
Model Predictive Control
CRUSHER
In a crusher circuit, the main challenge is to find a balance between the goal of the individual crushers and the overall circuit efficiency.
Challenges
- The balance between the individual crushers
and the overall circuit - Maintain circuit efficiency
- Ensure crushers are choked
- Crusher capacity vs. feed
- Equipment wearing
- Power consumption
MPC Benefits
- Maximize crusher circuit throughput
- Process stability (tons/h; size)
- Optimum energy efficiency
- Decrease equipment wear
- Less trips (over current, high level silos)

Potential Gains
Maximize crusher circuit throughput between 5-10%
Model Predictive Control
GRINDING
The optimum control of grinding circuit is challenging and presents a major opportunity for optimization and energy savings. The particle size delivered by the grinding circuit affects immensely the efficiency of the downstream processes.
Challenges
- Complex behavior
- Process disturbances between variables are non-linear
- Energy waste due to overgrinding
- Requires operators with lots of experience
- Balance between throughput and downstream constraints such as optimum particle size
MPC Benefits
- Maximized throughput rate
- Decrease energy / ton
- Increased stability
- Reduction in particle size variation
- Delivers grinding circuit stability to achieve throughput and grind size targets

Potential Gains
Up to 10% increased throughput and 10% on reduced
specific energy with a P80 variability reduction of up to 50%
Model Predictive Control
FLOTATION
Flotation is complex, non-linear process which has a multi-variable interaction with the likes of mass pull, reagent addtion and air flow.
Challenges
- It is a complex process and is not fully understood
- Still relies on operator control
- A small disturbance in one process variable propagates to the final process output
MPC Benefits
- Increased recovery
- Increased and stabilized cleaner grade
- Optimized reagent consumption

Potential Gains
Increased recovery of up to 3%, reduction of reagents
of up to 3%
Model Predictive Control
THICKENER
Thickener performance will have a direct effect on overall plant operation, however, many thickener installations operate below design limits and usually receive low prioritization around optimization.
Challenges
- Water conservation
- Optimum reagent consumption
- Little control and local knowledge
- Not often integrated into control system
- Conventional control is inefficient (long residence time, large disturbances and non-linear behavior)
MPC Benefits
- Increased underflow density
- Decreased flocculant consumption
- Increased overflow clarity
- Better water utilization

Potential Gains
Increased water recovery up to 3%, reduction of
flocculant of around 2%
Model Predictive Control
REFINING
Metal refining technologies face growing challenges associated with decreasing concentrate grade causing an increase in thermal inefficiency and lower productivity. Impurity concentrations are on average increasing, creating potential environmental threats and additional processing costs.
Challenges
- Final product under specified grade
- Change in feed characteristics
- Final product variability
- Temperature control
MPC Benefits
- Stabilization of the operations
- Improved quality
- Product consistency
- Energy savings
- Reduced emissions

Potential Gains
Up to 35% reduction in temperature variability (slag)
in copper smelter
Model Predictive Control
MATERIAL HANDLING
Material handling can be a complex task, and can be even more complex in operations requiring route management combining multiple conveyors.
Challenges
- Inconsistent production rates
- Loss of production at shift change
- Conveyor system trips
MPC Benefits
- Maximizing ore flow
- Operating to maximum constraints
- Minimizing equipment trips
- Extending equipment life
- Minimizing interruptions during shift changes
- Energy conservation

Potential Gains
20% increased throughput
Model Predictive Control
Click the diagram to learn more.
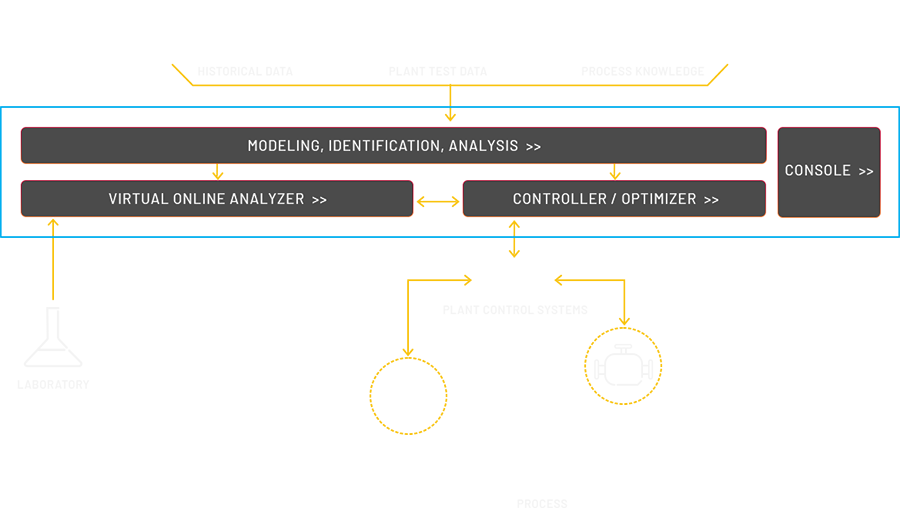
Model Predictive Control
MODELING, IDENTIFICATION, ANALYSIS
Hybrid modeling functionality allows the user to take advantage of all of the known information (empirical data, equations, equipment specification, etc.)
MODEL CREATION
DATA
MATRIX MODEL >>
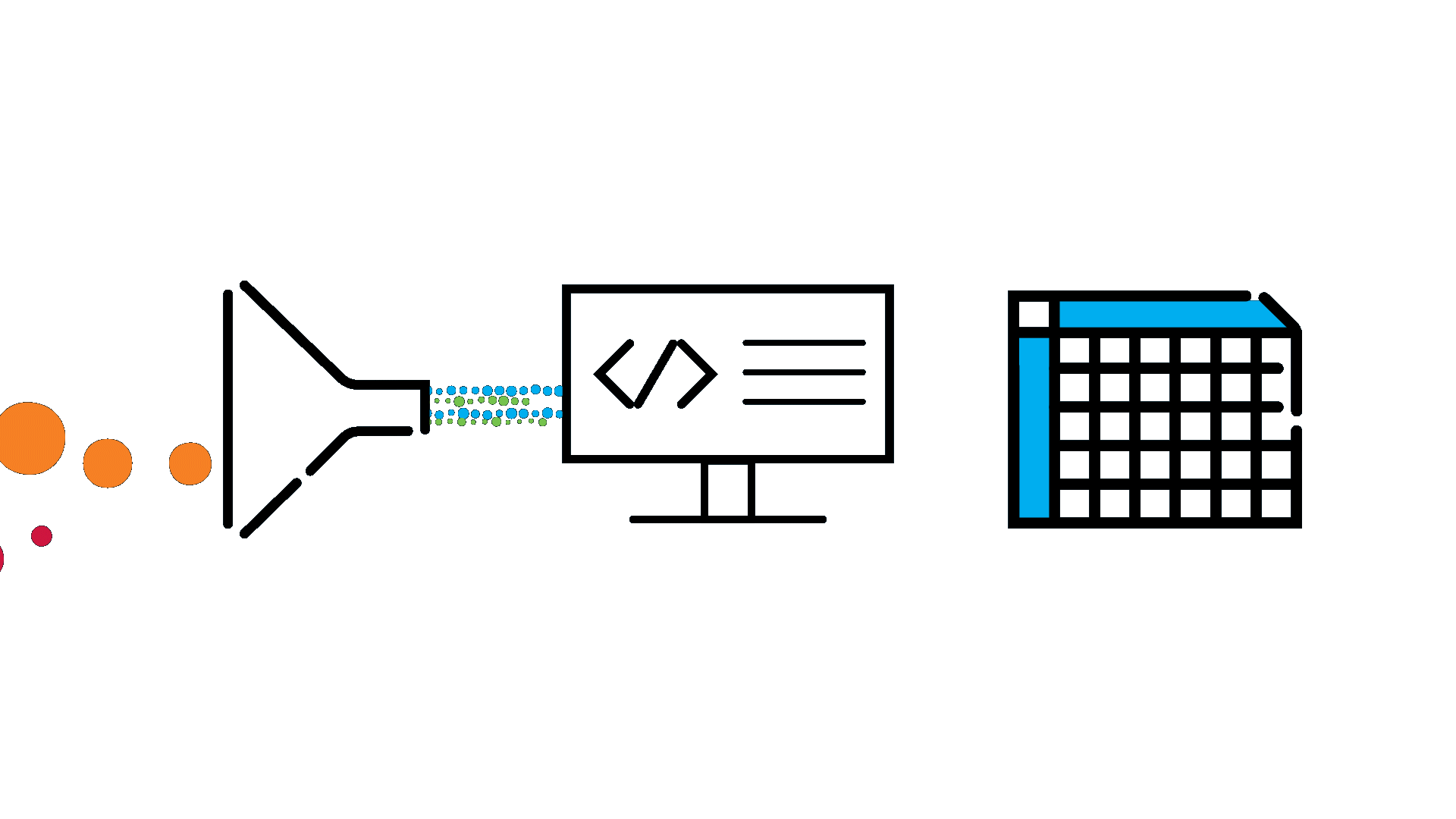
Controlled variables(CVs)
Process variables to maintain at a target or within a range (can be considered outputs)
Constrain variable (CCVs)
The state is forbidden to penetrate or may have physical limitations
Manipulated variables (MVs)
A manipulated input is one that can be adjusted by the control system or process operator
Disturbance variables (MVs)
Disturbance variables – these are also called “load” variables and represent input variables that can cause the controlled variables to deviate.
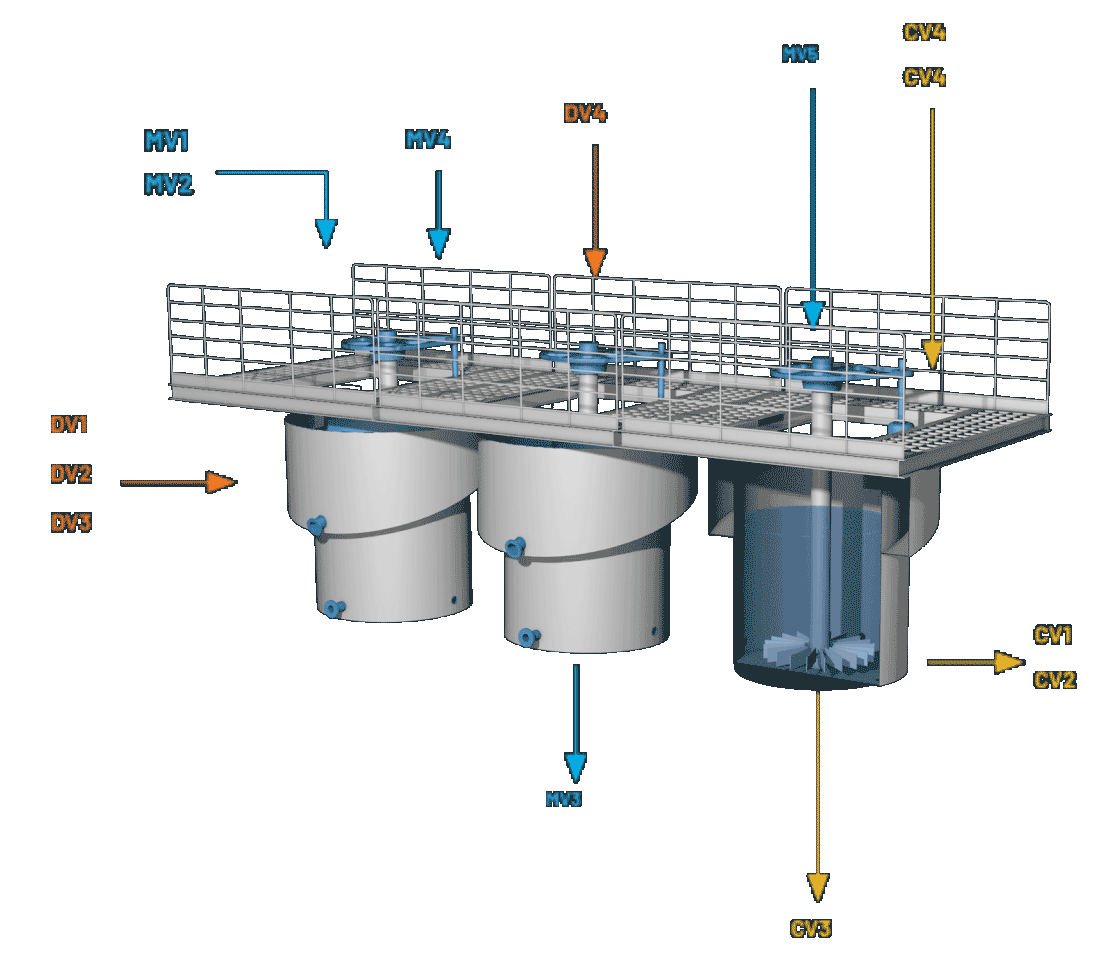
Model Predictive Control
MODELING, IDENTIFICATION, ANALYSIS
Hybrid modeling functionality allows the user to take advantage of all of the known information (empirical data, equations, equipment specification, etc.)
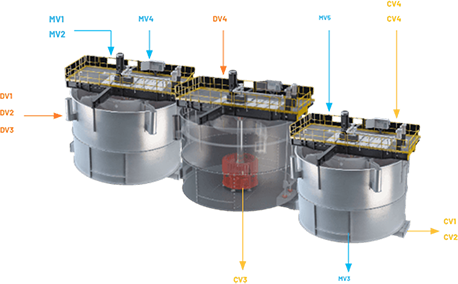
Model Matrix shows dynamic relationships between process outputs and inputs.
The system predicts future values of the outputs by movement of all the inputs (manipulated variables and disturbance variables).
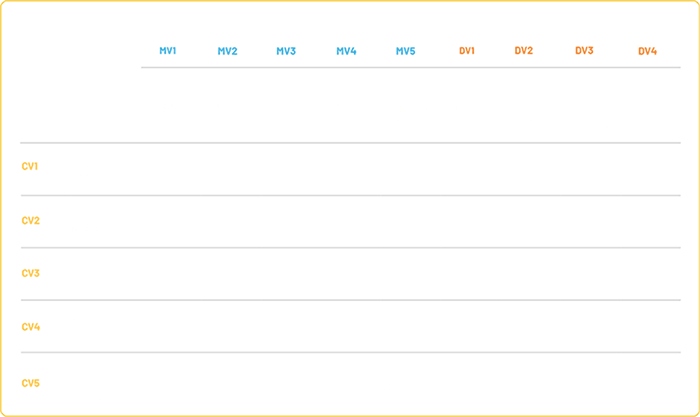
Model Predictive Control
VIRTUAL ONLINE ANALYZER
Virtual Online Analyzers replace sparse, or infrequent, feedback from lab or analyzer.
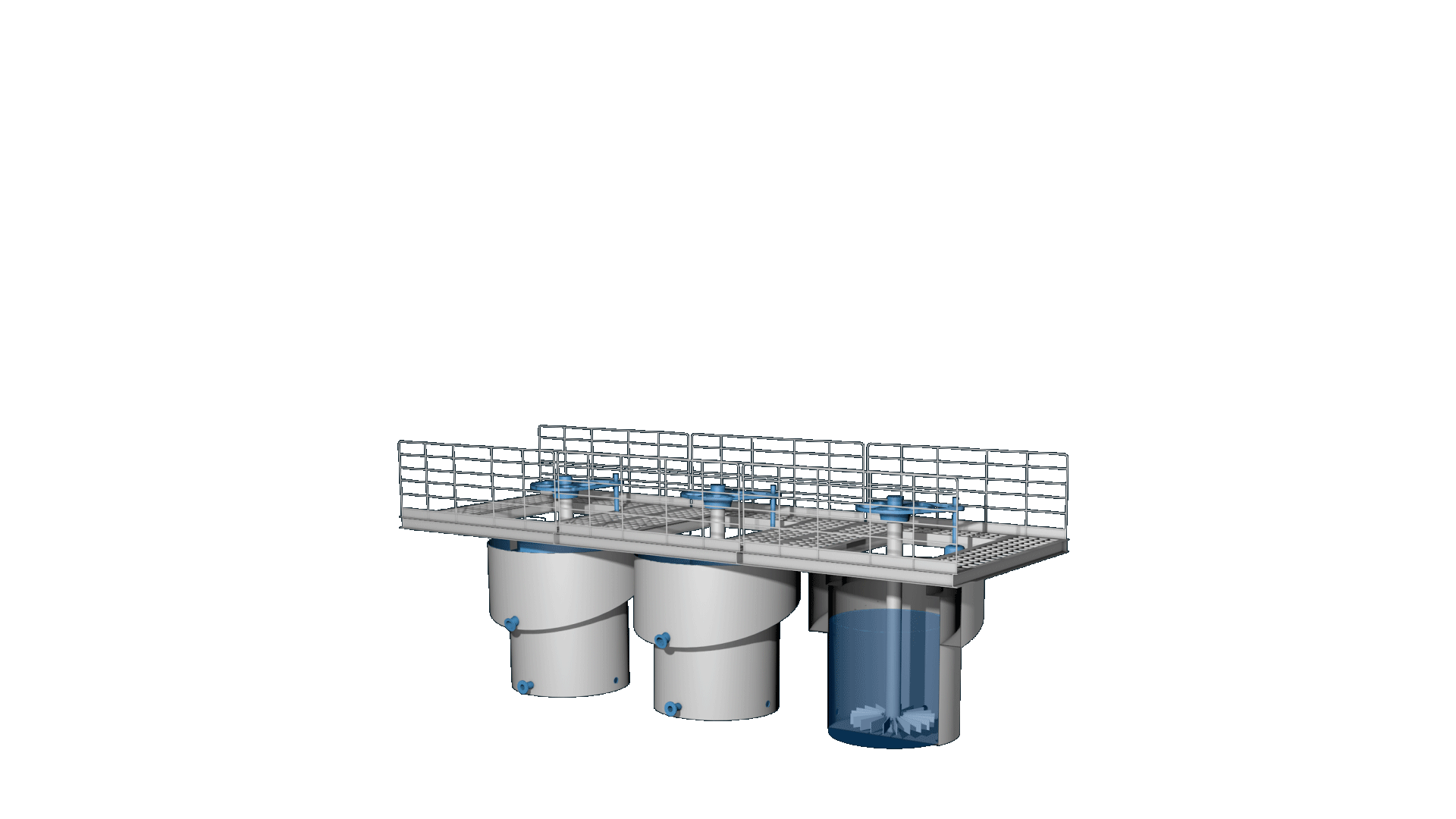
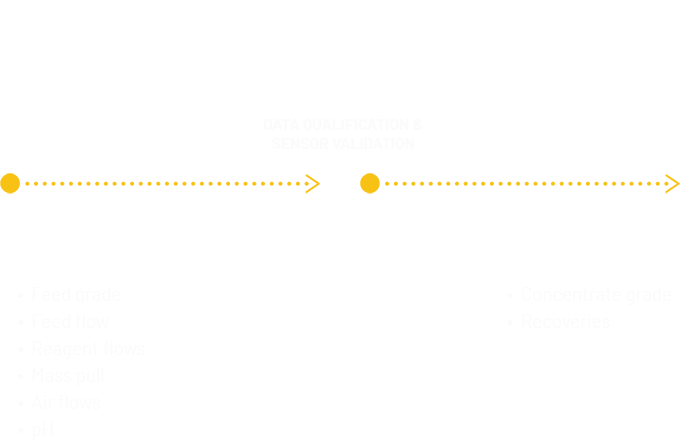
Model Predictive Control
CONTROLLER / OPTIMIZER
MPC uses a model of the process to predict how the process output variables will respond to changes in the process input variables and disturbances.
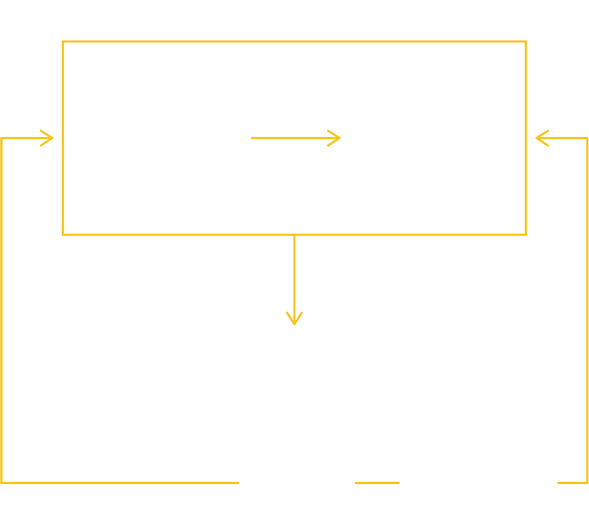
MPC allows for process stability while delivering peak performance since it finds optimal tradeoffs operating closer to system specifications limit whilst managing the process within constraints and safety margins.
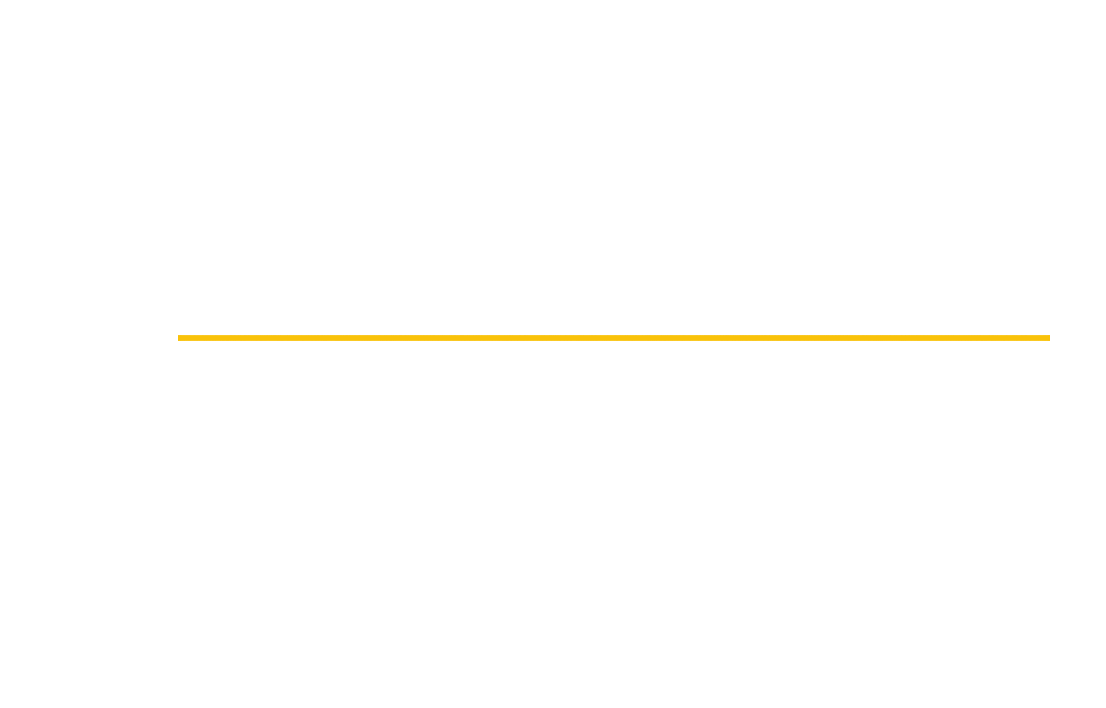
Model Predictive Control
CONSOLE
Built-in controller KPIs provide easy to understand views of control utilization, time at constraints, model error, and other key metrics to determine effectiveness of the control.
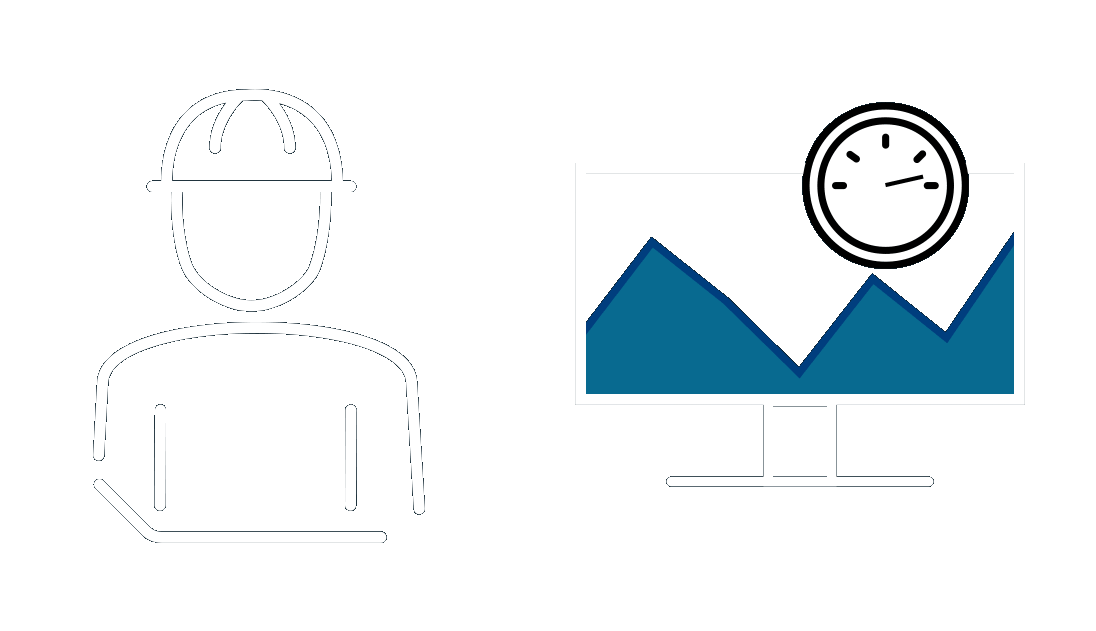
Additional metrics for production, quality, energy usage, and other factors can be easily configured to provide continuous measurements of the benefits derived from the application.
EXAMPLES
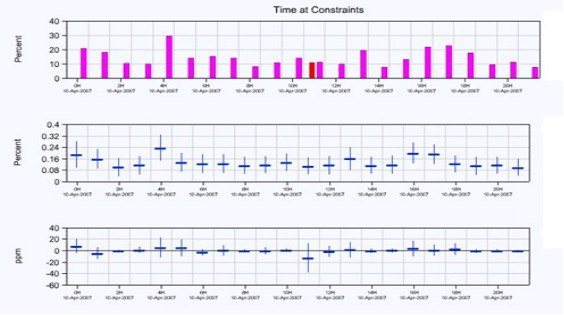
Variation
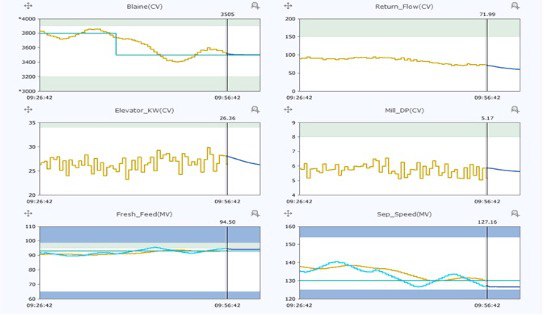
Process Trends
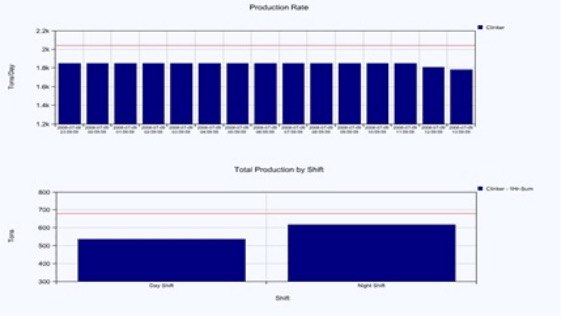
Production Rate
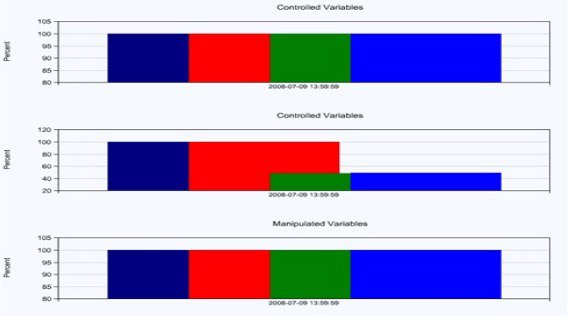
Control utilization